Just a quick post as I’m musing the consequences of what could be a fundamental shift in AI.
First, the background
OpenAI is releasing a new model called o1 or Strawberry. More from The Verge:
The training behind o1 is fundamentally different from its predecessors, OpenAI’s research lead, Jerry Tworek, tells me, though the company is being vague about the exact details. He says o1 “has been trained using a completely new optimization algorithm and a new training dataset specifically tailored for it.”
The main thing that sets this new model apart from GPT-4o is its ability to tackle complex problems, such as coding and math, much better than its predecessors while also explaining its reasoning, according to OpenAI.
Also this from Shelly Palmer:
o1 models handle longer context windows, enabling more comprehensive text understanding and generation. This capability allows for processing larger documents, making them valuable for extensive data analysis and document summarization. However, these enhancements come at a cost. The new models require significantly more computational resources, with operational costs estimated up to 10 times that of GPT-4.
Why this matters
This looks like a fundamental change in the business model for AI. Most software operates on a large upfront cost, then sold to as many users as possible.
In the good old days (i.e. last week), this was AI. You spend lots of money training and fixing, but the inference part (i.e. queries from users) was relatively small. Think of it like a regression – you spend a lot of time and effort getting to the answer that y = 2.1x + 5.3 and then you spend some more time and effort working out that a slightly better model is y = 2.2x + 5.2. But, when it comes to people using your equation, the calculation cost is tiny.
If o1 is a precursor to what is coming in the next generation of models, that business model no longer stands. Check this illustrative chart from X and DrJimFan:
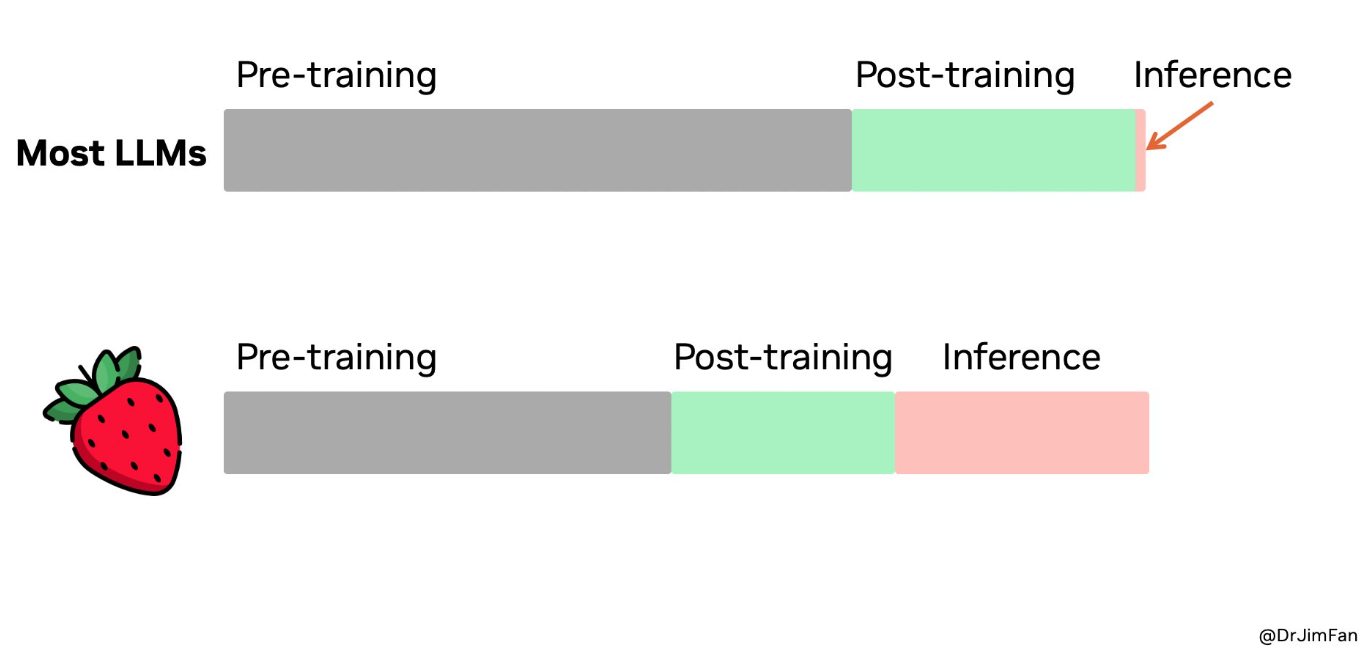
What does this mean
Now, let me start by saying that when it comes to AI, we all have a small amount of data extended out to infinity. Small changes in growth rates and starting conditions can lead to radically different outcomes. So take all this with a huge disclaimer about the faith in forecasts. And the dangers of extrapolating short trends out forever.
More spending on chips? Absolutely.
What chips? It is less clear.
It seems highly unlikely that we will go back to old technologies – CPUs (the domain of companies like AMD or Intel) do not look like making a resurgence.
GPUs (the domain of NVIDIA) seem quite likely to be a winner in that they are very good at the training and pretty good at the inference.
There are some question marks about whether a new class of chips will emerge like Groq that will become a new standard in the inference part and take market share of the inference away from NVIDIA.
Which model dominates? Do people want “pretty good” answers for free or “excellent” answers for a lot of money? It depends, obviously, on the application. I’m guessing self driving needs excellent, as do most engineering applications. Search, social applications will probably opt for “pretty good” and free. But who knows?
Might other models emerge which are better? Yes. They absolutely will. Maybe the next generation will be back to large training costs and low inference costs. This is all speculation.
Change in business model? If compute costs are high, then do providers need to change business models? Or at least switch to a usage-based one where maybe the users making lots of queries are charged a lot more. If so, the AI is a lot less likely to be “winner takes all”. Why? In the low ongoing cost model, early winners have all the users and make lots and lots of money, then keep spending that money to stay ahead of everyone else.
For example, at the start, say it takes $1b to set up a model and $10m a year to run it. Lots of companies can try, and startups abound. But in a few years, it will now take $25b to set up a model and $50m a year to run it, so the number of companies and start-ups will fall dramatically.
Compare that to a model where it takes $5b to set up a model and $2b a year to run it. The start-up cost is a lot more accessible, and groups of customers can band together to support new entrants. Big users might be more likely to start their own model.
Margins are probably lower, especially if there are more entrants.
Repeat Disclaimer!
This is all really early days, inference and speculation.
I’m quite interested in other implications I might have missed… hit me up in the comments.
————————————————-
Damien Klassen is Chief Investment Officer at the Macrobusiness Fund, which is powered by Nucleus Wealth.
Follow @DamienKlassen on Twitter or Linked In
The information on this blog contains general information and does not take into account your personal objectives, financial situation or needs. Past performance is not an indication of future performance. Damien Klassen is an Authorised Representative of Nucleus Advice Pty Limited, Australian Financial Services Licensee 515796. And Nucleus Wealth is a Corporate Authorised Representative of Nucleus Advice Pty Ltd.